AI data management is the process of formulating, putting into action, and overseeing the management of policies, strategies, and programs that govern, protect, and improve the value of the data that is collected by an organization.
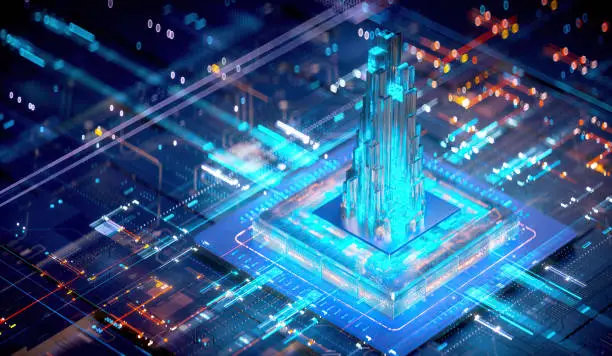
The data management framework is concerned with the entire data lifecycle management process and how it can be optimized to assist in guiding strategic operational decision-making. This is because the framework is designed to help guide decision-making.
A comprehensive AI data management plan makes use of a variety of data management tools and data management techniques. These data management tools and techniques include, but are not limited to, data architecture, data governance, data integration, data modeling, data security, data warehousing, master data management, as well as business intelligence and analytics.
Business intelligence systems evaluate and transform complex data into meaningful, actionable information, which can be used to support more effective strategic, tactical, and operational insights and decision-making. These systems combine data gathering, data storage, and knowledge management with data analysis.
AI data management process
The first steps in the data management process are the design and deployment of a data architecture, the generation, processing, and storage of data in a data repository, the integration of data into a data warehouse or data lake for further analysis, the performance of data quality checks to identify and resolve errors via data cleansing, and the creation of data governance to ensure that data is consistent.
The significance of AI data management
When correctly handled, data has a tremendous amount of potential. The management of data guarantees that the data are located in the appropriate location, at the appropriate time, in the appropriate format, and that the use of the data is managed for all users.
It is possible to utilize managed data that is of a high quality, consistent, and compatible to make informed business choices and reports, optimize company operations, save expenses, promote sustainable business development, and enhance overall operations management using data that has been managed.
Unmanaged data may result in incorrect conclusions, may cause data environments to become chaotic, may expose enterprises to the risk of data breaches, and may violate regulatory compliance requirements relating to data privacy and protection legislation.
The benefits of having a data catalog driven by AI
Data catalogs are more important now than they have ever been before as a result of the growing volumes of data that organizations own. A data catalog is a location where various stakeholders may store and exchange metadata about all of the data assets that come from a variety of data sources.
Metadata is information that describes other information, such as where the data is kept, who the owner is, what its categorization is, and certain statistical properties of the data. Any analyst, developer, or data scientist who is seeking available data assets to employ in their project should look in the data catalog. This is the appropriate location for them to look.
Automation and cooperation are the two primary pillars upon which a successful data catalog is constructed. Users get additional information about an asset as a result of automation, which guarantees that the technical metadata is enhanced with business metadata. Because not everything can be automatically enhanced, it is essential to offer a user-friendly interface so that people may enrich certain information manually, debate it, and share the knowledge they have gained.
How AI can help in making data more reliable
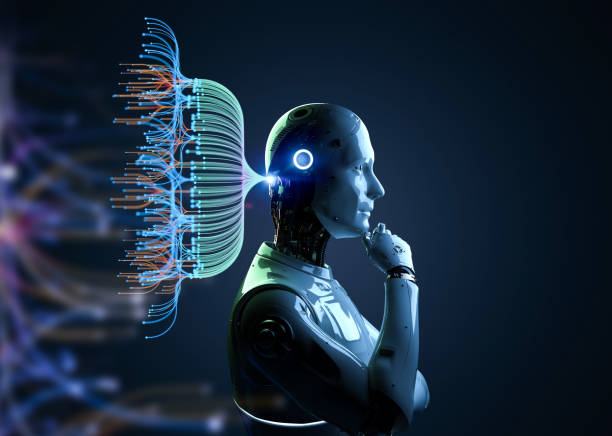
AI is the appropriate tool for data quality management (DQM) because within most business models, it is the only solution that can handle the amount and complexity of data necessary without blowing your IT budget. This makes AI the ideal instrument for data quality management (DQM).
In addition to that, AI has the potential to have a direct influence on some of the most important aspects of data quality, including correctness, completeness, dependability, and relevance. The development of each of these areas takes a significant amount of study, which is something that AI is capable of doing on a larger scale and at a quicker speed, not to mention at a lower cost, than an army of analysts.
However, in order to have a complete understanding of why AI is the greatest tool for DQM, we must first comprehend the reasons behind why DQM presents a one-of-a-kind multidimensional difficulty.
What does effective AI data management need?
More accelerated creativity and achievement of outcomes
In order to get quicker results, you will need to integrate all phases of the AI data lifecycle, as well as condense mixed data and protocols.
Achieving more with less resources
The skills gap in the IT industry is widening at an alarming rate. You need to process data relating to AI more quickly, and you also need to automate the processing itself.
Adaptive scaling
You will require scalability in order to manage volume and methods to decrease the overall amount.
Conclusion
The connected society we live in today is causing an explosion of data on a scale never seen before in corporate companies. It is not simply the quantity of data that requires quick attention. Rather, it is the diversity of data coming from more recent sources, such as sensors attached to devices that are connected to the Internet of Things (IoT).
In addition to that, the development of cloud computing technologies has provided the impetus for a shift in the focus of technology budgets, which have shifted from a concentration on the acquisition of hardware and infrastructure to one that emphasizes the use of technology and services in order to get the most out of the company’s data assets.
Because business ecosystems are becoming digitized at an increasingly fast rate, companies are having to deal with data that is quickly expanding and changing in relation to their goods, customers, suppliers, workers, and other stakeholders. In order for businesses to achieve success and get an advantage over their competitors, it is essential for them to be able to have effective AI data management.